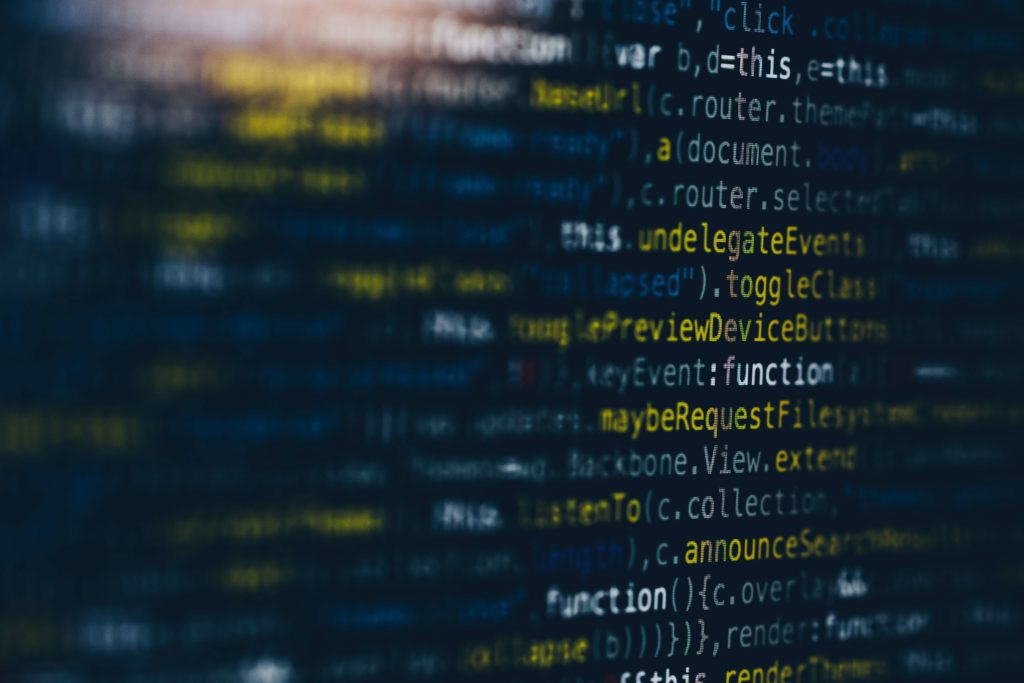
Is xAI the solution to the “black box” in machine learning?
We are in the middle of a revolution: airBnb has revolutionized the way we look for accommodation on our holidays; Uber has transformed the way we move around within cities; Amazon, the way we shop; and Netflix has transformed the way we watch TV.
Artificial intelligence algorithms are fed with metadata (in the case of Netflix: the genre, category of the series/movies, actors, crew, release date) and also by data associated with the behaviour of the users (playback time, searches they have made, rating of the content, device they have used, time they have watched that content…). With all this information, they are able to detect similar viewing patterns, showing certain content to specific user profiles.
Artificial intelligence algorithms
Although nobody knows 100% how Netflix recommendation algorithm works, on their page they offer a fairly detailed description of the system they use. However, they do not give what their “secret formula” is.
AI algorithms are usually like “black boxes”. We know little or nothing about their inner workings and this makes us often unable to explain their operation. And although it may not matter how these algorithms are able to offer us valuable content. Other algorithms do have a more direct influence on our daily lives.
AI brings great benefits both to companies and to the final consumer, as long as we understand how the decision making process is carried out.
Difficulties of traditional models with automatic learning
As a general rule, we can say automatic learning models (i.e. AI algorithms that learn by example), will improve their performance with respect to traditional models. They are much more agile in detecting complex non-linear patterns and interactions.
Traditional ai techniques will rely on human programmers to analyze how variables interact and, as a result, make decisions that provide a concrete result.
When designing an algorithm that follows a traditional model, anyone can include their own biases in the algorithm.
In the case of applying modern automatic learning models, predictions and decisions are faster and need little human intervention. However, this does not mean that this type of learning model is free of challenges. A model is trained with a data set. In some cases, this data may not represent the population for which it is designed.
When we understand and analyze a data set, we can see the behavior of different population typologies, thus we can detect niches and biases.
To which sectors can explainable AI (xAI) be applied?
One of the sectors that obtains the greatest benefit from explainable AI is the financial sector. Let’s imagine the process of granting a loan, the algorithm will be in charge of analyzing the applicant’s repayment capacity in a totally impartial and transparent way.
For this reason, it is essential to have a company that knows how to deal with bias, otherwise we would be generating an algorithm that would show undesirable results according to different discrimination parameters.
What is explainable AI and what is it used for in the banking sector?
That an automated intelligence is explainable means that it understands how and why the algorithm makes decisions and/or makes predictions. It also has the ability to justify the results it produces being global or local.
Global explanations will be in charge of describing the behaviour of the algorithm in general. In the case of bank loans it would serve to show a high rate in cases of people who are going to have difficulties in assuming that new debt.
On the other hand, local explanations will describe the behaviour of the algorithm in specific cases.
With explainable artificial intelligence we have to be able to explain what decisions the algorithms make, knowing also that they are made in an impartial way.
Counterfactual predictive models for xAI
When counterfactual predictive models are applied, we will be able to explain in the banking sector why the model rejects a customer’s loan application. We explain in a simple way how it works:
- We create a “twin” with the customer’s profile including: age, transaction patterns, etc. Then we make small variations of the values that define these variables (such as making estimates that decrease the proportion of income and expenses).
- In order to approve the loan based on one of those alterations, we then subject that “twin” to these variations repeatedly.
The data obtained during this process will allow us to identify the variables that justify the model decision.
On the other hand, the creation of these “twins” allows us to offer advice to the user to improve his financial health making it easier for him to obtain a loan in the future.
Bank advisory services: how does an AI help?
As we have already seen, AI algorithms carry out predictions of a client’s income and expenses. We can use the algorithm to recommend products that best fit a customer’s profile and needs. We can even use the algorithm to give advice to increase a customer’s financial solvency.
Let’s look at Netflix for example. In the case of recommending a bad movie, you will lose two hours of your time. However, when we talk about financial products, the risk is much higher. A mortgage can last up to 35 years.
Does explainable artificial intelligence (XAI) open black boxes?
Without a doubt, there are countless applications for the XAI:
- Improving the standard of living through sustainable investments.
- Planning opportunities for financial institutions and ngos
- Among many others.
In short, X.AI is not only a useful tool for opening the black box of an AI algorithm, but it offers an unparalleled opportunity to increase transparency and fairness.