A data lake is a centrally operated data storage repository. In this article, we talk in depth about how a data lake works and how to choose the architecture of a data lake.
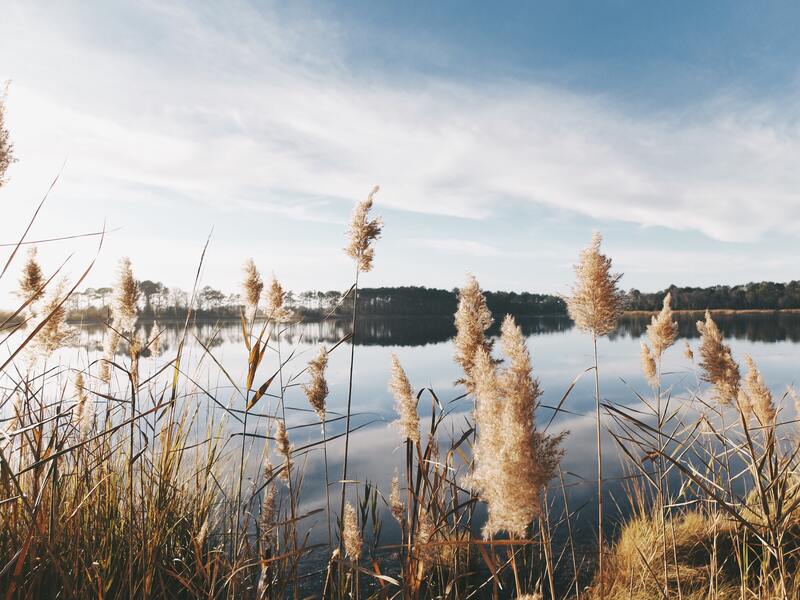
What should the architecture of a data lake look like?
Organisational objectives
Before defining the data lake structure, it is important to establish and define the objectives to be achieved. The data policy to be implemented must be clear.
It is possible that at the moment of implementing the Data Lake not all the answers are clear, for this reason it is also important to choose scalable options that can be adapted to the needs that arise over time.
Of course, this is a technology that requires an experienced team for its implementation and where experience and trial and error will be very important to achieve good results.
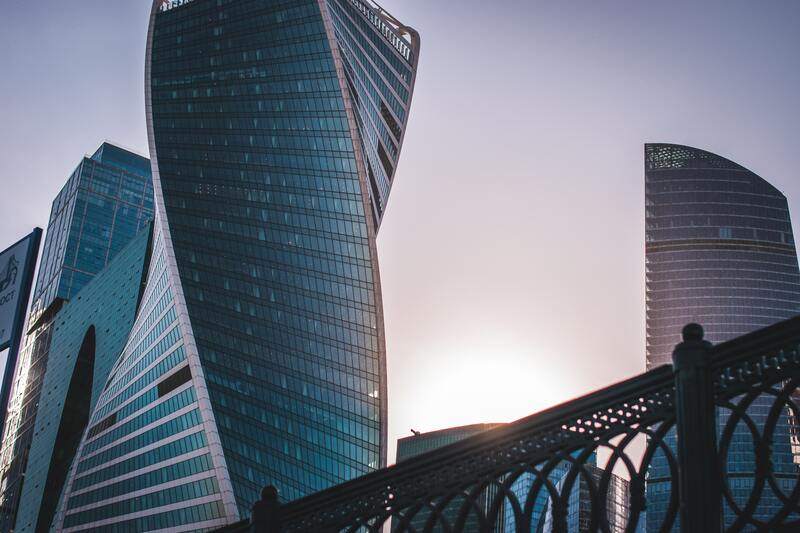
Steps to choose the data lake architecture
Once the objectives and the data policy have been defined, there are other aspects to be taken into account
- Establish the final platform
- Establish a data fprofile and data cataloguing.
- Perform and establish measures such as backups and archiving of data.
- Ensure traceability and consistency of data.
- Establish layers within the data lake for each user and their respective skills and capabilities.
- Establish data governance
- Develop and establish a data security policy.
- Incorporating automation, AI and machine learning capabilities is essential to improve performance and results.
- Incorporate the DevOps concept, i.e. the integration between development and operations.
- Administration, establishing the owners (users), through some metadata solutions.
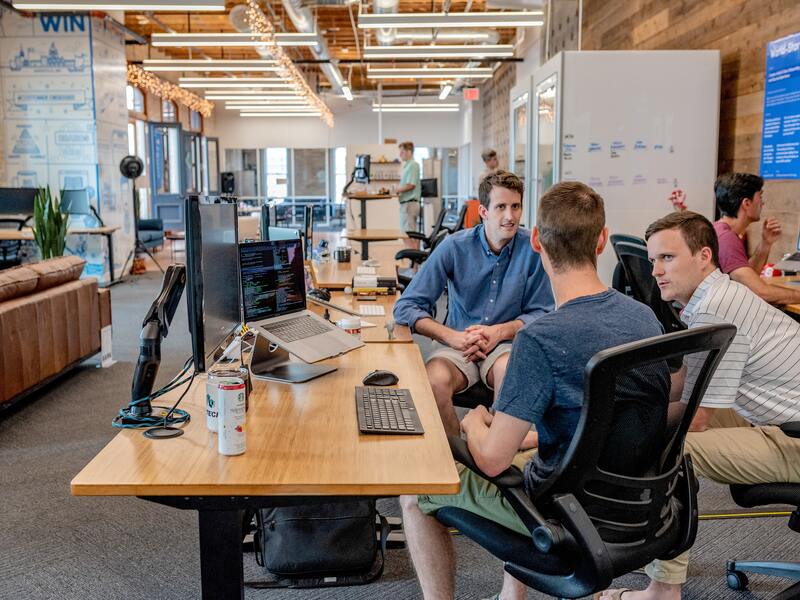
A highly recommendable option is to start with scalable data lakes, as is the case of our data lake in-a-box.
If you are interested in knowing how OpeSistemas works with the Azure data lake, don’t miss this article.
As we can see, it is essential to establish clear criteria to achieve the best performance of this technology.