DATA
Industrialize your data practice and scale
Automation | Governance | Culture | Knowledge Graphs
Automation
Our experience tells us that machine learning models can be developed and implemented relatively quickly and easily, whereas maintaining them over time is complex. In other words, there is a major qualitative leap between the most experimental phase – the first models, produced almost as proofs of concept – and a maturity phase with complete mass production of the lifecycle of the models which are moreover already embedded in an organisation’s business processes.
The technical debt in this type of ML-based system compared to traditional ones has to do with the data’s enormous impact on the system’s behaviour (data drift) and the need to work on complex distributed architectures and a great range of technologies in production (data pipelines and lineage, experimentation, feature engineering, flow monitoring, numerous technologies for automation, etc).
At neuroons we are specialists in the tools, frameworks and practices that allow us to automate that lifecycle to conduct machine learning at scale:
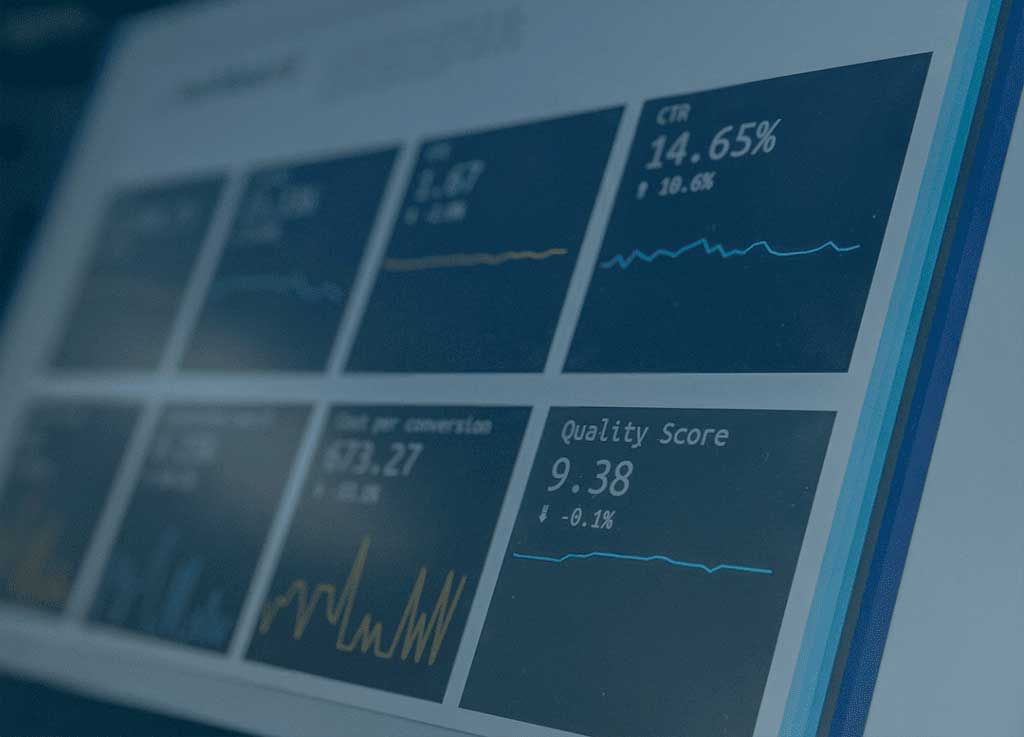
01
Reproducibility and traceability of data experiments
02
Simplification of modelling with automation and AutoML architectures
03
Maximum reuse with feature stores
04
Operationalisation of the models in scalable infraestructure and with A/B testing support
05
Model supervision to detect concept drifting
06
Automation of the lifecycle with the new tools for MLOps
Governance
Only half the organisations and companies we work with have formal processes in place to manage their data at the corporate level. The rest range from ones with tactical strategies at the departmental level to those with no initiatives at all.
One of the stumbling blocks we have identified at neuroons is the difficulty of justifying outlay on an initiative which does not have an immediate impact on business performance. This is even more the case if there is no regulatory pressure due to the kind of data handled.
At neuroons we deliver pragmatic approaches to this problem by using proprietary and open source technology with a graphical design which can be easily integrated into current data architecture to monitor responsibility for the data and their lineage.
Culture
Our years of experience working in companies which implement data-driven practices have enabled us to see at first hand numerous approaches to successfully becoming data-driven. This gives us insight into how to address cultural change and which dimensions need to be worked on and nurtured.
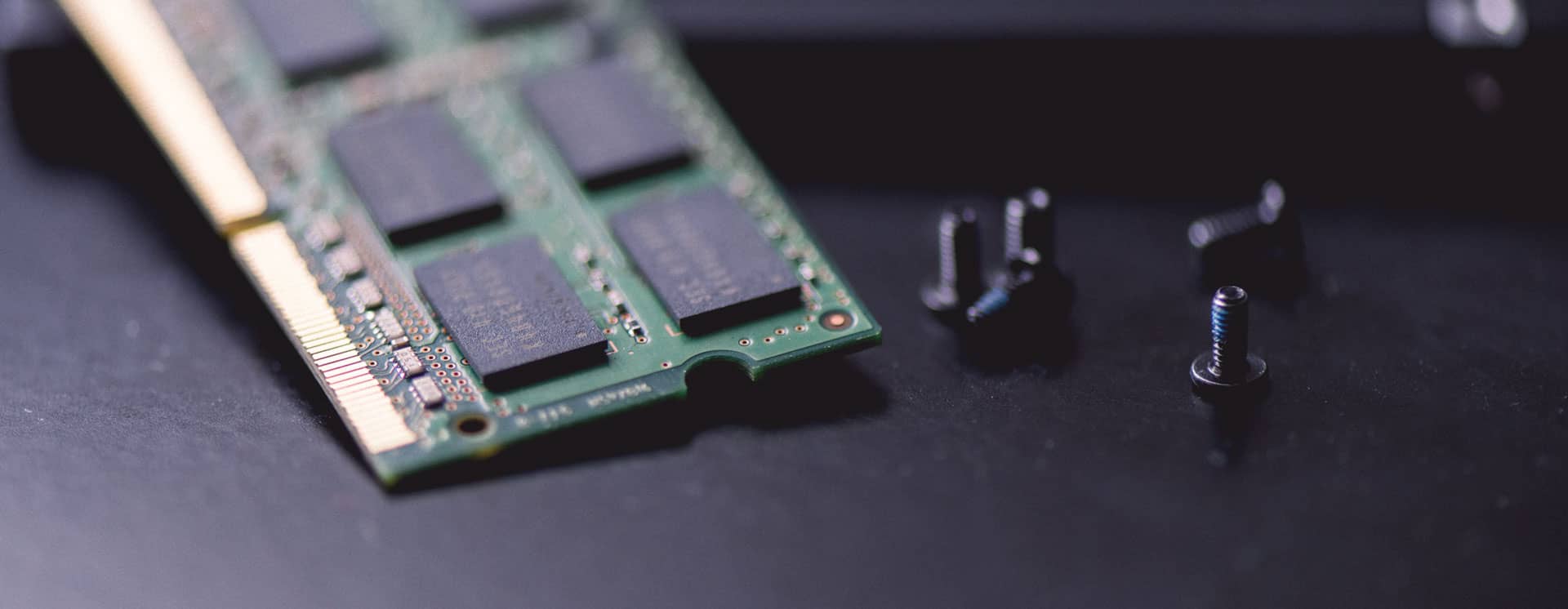
At neuroons we can bring that experience to bear on several fronts for change management:
01
Assessment
02
Organisation for data
03
Technology strategy
04
Communication and Marketing strategy
05
In-house training and attracting external talent
Knowledge Graphs
Fraud prevention analyses using more traditional approaches and technologies have a very high computational cost and the first consequence is extremely poor performance by all associated management processes.
The best method is to classify the available data by knowledge domain and then model and depict the available information for each of these domains in graphs. It is easier and cheaper to identify and code abnormal or fraudulent activity patterns on such graphs. And ultimately machine learning algorithms can be used to classify behaviour and even predict future behaviour.
We are specialists in graph-based analysis and in using machine learning algorithms to classify and predict abnormal behaviour. We can apply these capabilities to areas such as cybersecurity, preventing financial crime, money laundering, etc.
Use cases
We are a value-based company that believes in sharing, collaboration and respect. We are passionate about open source, forefront technology, communities and technical knowledge. Working with us means working in a caring and open environment.